Dr. Alex Papalexopoulos, Ph.D.
I. INTRODUCTION
Over the last 10 years a combination of policy directives and technological advances has led to a rapid and significant addition of Distributed Energy Resources (DERs), such as Renewable Energy Sources (RES) generation, Demand Response (DR), Storage, Electric Vehicles (EVs), into the distribution grid and Behind the Meter (BTM) in many power systems around the world. At the same time the IoT revolution has emerged as a major market force that is expected to create massive flexible capacity which can be harnessed to solve grid problems and assist in the integration of RES into the grid. The penetration of DERs across various levels of the power system has created a hierarchical market structure where DERs could participate, individually or through aggregations, in the form of Virtual Power Plants (VPPs) in BTM markets, distribution and wholesale markets. The landmark FERC Order 2222 has further created a high-level regulatory framework for allowing DERs to bypass the distribution grid and directly participate in wholesale energy markets. In this multi-tier market architecture, TE markets are emerging by allowing customers either as individuals or in aggregate, to actively engage in energy markets by negotiating and responding to “value signals,” based on price, demand, time of day, and other grid and local market considerations.
This paper highlights and advocates a level-playing field for DERs where their services can be valued in multi-tier markets in a way that distribution markets, operated by Distribution System Operators, (DSOs) are not subjugated to the Independent System Operators or Transmission System Operators (ISOs/TSOs). In practice, in most ISO/TSO markets, which have been in operation for more than 20 years, Day-Ahead Markets (DAM), Intra-Day Markets (IDM) and Real-Time Markets (RTM) are running in sequence during the day with different frequencies and time horizons and produce market prices, schedules and dispatch levels for all resources participating in the transmission level market. In some market implementations capacity markets have been developed to ensure reliability and security of supply. These markets have matured, even though certain modifications are necessary, are not the focus of this paper.
This paper focuses on the remaining supply chain of downstream markets that are currently under development and implementation. It presents a design of organized Nodal Local Energy Markets (LEMs) at the DSO level where all distribution constraints from radial or meshed unbalanced distribution grids are enforced. The proposed market architecture for organized DSO markets puts a premium on the locational marginal prices at the distribution level. It also presents a DER optimization platform for aggregating smart devices and other DER low voltage assets, based on distributed stochastic control and creating VPPs for market participation in all levels, including the TE market. The LEM market design and the DER aggregation platform of BTM assets are the two missing elements to make the multi-tier market a reality. Finally, the paper presents a TE trading model coupled with the Blockchain technology and the use of a digital currency for settlements.
II. THE EMERGENCE OF THE HIERARCHICAL ENERGY MARKET ARCHITECTURE
The hierarchical energy market structure (see Figure 1) affords the opportunity to individuals, or local groups such energy communities, to form LEMs, which are distributed in nature, to address economic and environmental opportunities, and local energy needs often in response to regulatory incentives. DERs enable consumers to become prosumers and provide flexibility and other services to the grid. A LEM may consist of a small number of market players close to each other, or it can comprise of a wide geographical region, town or a municipality. A LEM community generates and consumes energy locally and may or may not be connected to the main grid. This architecture is expected to provide a well-coordinated series of temporally and spatially linked markets. Simultaneous DER participation in these markets should be voluntary based on the decisions of the DER owners to maximize their value to the grid.
The local market platform is expected to clear the market in the distribution level based on bids and offers from DERs, produce market prices and solve specific grid problems. The presence of distribution network constraints further creates the need for Locational Marginal Prices at the distribution level. This evolving environment, which puts substantial value on the locations of the DERs can be materialized by a DLMP-based LEM market; such a market is intended to procure competitively grid services for DSOs while maximizing the value of the DERs in the system and enhancing their integration at the edge of grid.
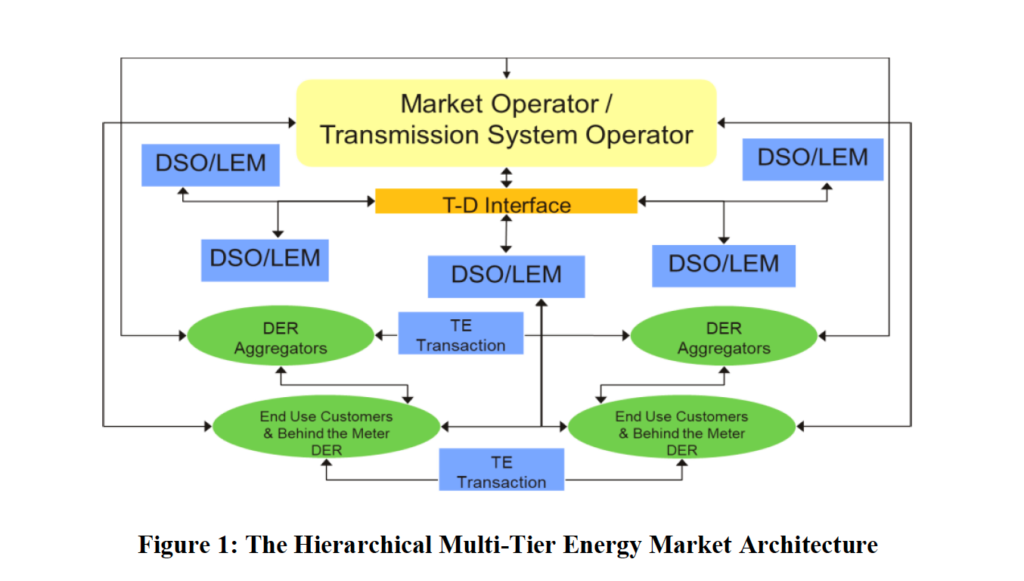
The fundamental objective for this market architecture to scale is to ensure that all regulatory or market barriers are removed so that DERs can freely participate in all markets of their choosing, ISOs/TSOs, LEMs/DSOs, BTM and TE markets. The BTM market development takes advantage of the IoT revolution and the massive penetration of edge grid assets close to the customer. The proposed hierarchical market architecture enables the development of TE trading markets by providing grid edge devices the opportunity to interact with other devices in a trustless blockchain environment, while locally optimizing operational decisions based on local market conditions and user preferences and taking into account grid reliability constraints. As the TE markets evolve and mature, it is expected that the ISO/TSO and LEM/DSO markets will be simplified and more products and services will be developed from the bottom up taking advantage of the automated responses from DER resources with ex-post settlement.
III. THE NODAL DSO LOCAL ENERGY MARKET
In this Section we present the market design architecture we have implemented for the evolving LEM/DSO energy market in the distribution level. We advocate a DSO market which is not subjugated to the wholesale competitive ISO/TSO market. We take exception to efforts to deploy market prices produced by the ISO/TSO augmented by estimates of the cost of congestion and losses in the distribution system. Depending on the assets participating in a LEM, namely the generation and the load units, the clearing optimization problem can either be formulated as an LP or a MIP problem. In the MIP case, DLMP prices are still produced from an LP solver that is executed at the solution of the MIP optimization solution in a post solution pricing run with the integer variables fixed at those values calculated by the MIP solution.
The proposed LEM/DSO market architecture relies on offers/bids submitted to a LEM market platform which is operated by the DSO. Wide applications are still lacking because implementations are riddled with market and technical challenges, such as lack of accurate data in distribution, unbalanced distribution networks and radial networks coupled with a lack of a substantial number of DERs along each feeder required to solve specific distribution constraints. The lack of substantial market depth creates challenges in producing well behaved DLMPs but as the volume of DERs increase, this problem is expected to be effectively addressed. Our proposed LEM market platform is based on a Security Constrained Unit Commitment (SCUC) overlaid on a fully coupled unbalanced AC Distribution Power Flow (DPF). The SCUC algorithm is based on MIP based optimization technologies, similar to the ones deployed over the last fifteen years to clear wholesale ISO/TSO energy markets. In general, the resulting market DLMP prices are produced similarly as in the wholesale market (but in this case for each phase of the distribution grid) using the shadow price of the power balance equation (the energy price component), the shadow prices of congested lines and the Power Transfer Distribution Factors (the congestion price component) and the loss sensitivities in each node (the loss price component). The iterative LEM optimization process is illustrated in Figure 2.
The optimization problem takes into account the physical constraints (usually distribution line constraints and voltage constraints) imposed by the DSO. The LEM operator solves a SCUC problem which determines the resource commitment status, the energy schedule and prices and provides data to settlements to settle energy and other products, or other uplift payments or charges. The resources that submit offers and bids to the LEM platform could be various aggregations of IoT smart devices, DERs, such as RES, EVs, Electric Storage Resources (ESRs), DR or other distributed generation assets.
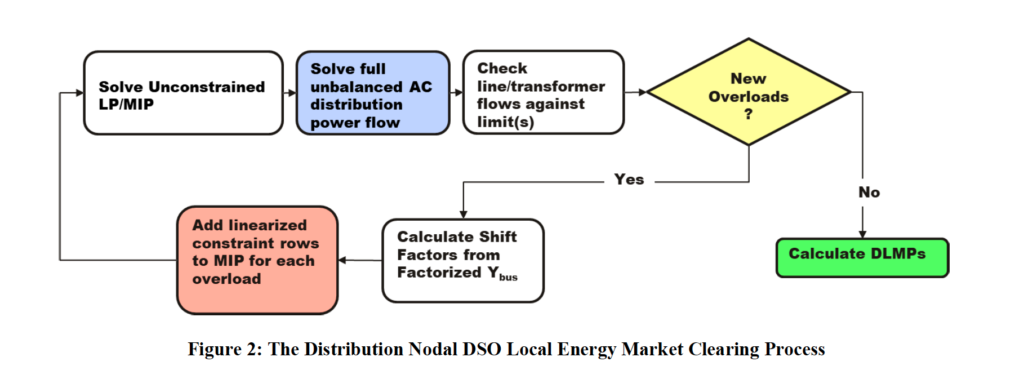
The DAM clearing problem takes as inputs next day DER generation offers, load bids and bilateral transactions schedules. The RTM formulation is similar to that of the DAM. The time interval of the optimization is one hour for the DAM and 5 minutes for the RTM. The objective function minimizes the total procurement costs subject to device, system and grid constraints. The constraints in the optimization are as following: a) the power balance constraint, b) the distribution constraints, c) the voltage constraints, etc. Our implementation accommodates a flexible bidding format structure consistent with wholesale energy market designs, including simple “price-quantity” offers and bids, block offers, different types of complex block offers, which are prevalent in European-style markets, and bilateral transactions. Slack variables with penalties are used to provide a relaxed solution where insufficient resources are available to meet constraints.
The distribution grid constraints are presented as linearized functions of the nodal power injections around the base operating state using the Power Transfer Distribution Factors (PTDFs) (i.e., the Shift Factors from the factorized Ybus admittance matrix) in the Three Phase Unbalanced AC Distribution Power Flow (DPF) problem. To enhance the performance of the proposed optimization algorithm only a subset of distribution lines is passed from the AC distribution power flow application to the SCUC algorithm. To avoid oscillations in the SCUC-DPF iteration process, circuits are added into and never deleted from the critical set. The inter-temporal constraints include the storage related constraints of maximum and minimum storage levels and taking account of the initial storage state of batteries and specifying daily energy limits for certain resources. The bus admittance matrix only needs to be formed and factorized once for each network. The Voltage Constraints are represented in the linearized formulation around the power flow solution. The clearing of the optimization engine produces the DLMPs for each bus and phase.
The full AC DPF application uses the sparse Ybus Matrix Iterative (also known as the Implicit Zbus Current Injection method) to solve for the voltages and phase angles, transformer tap ratios, capacitor status and solar/battery inverter reactive power for a given set of loads and distributed generations. The implemented algorithm is commonly used in distribution networks because of its superior convergence properties with the highly radial network with high R/X ratios that are normally found in distribution feeders. The traditional Newton Raphson methods used for the transmission power flow (used in the solution of the wholesale energy market clearing) do not normally work well on radial distribution feeders widely present in DSO markets. Open issues related to the coordination between the DSO/LEM and ISO/TSO remain, including communication protocols in all timeframes in both the operational and planning phase conflicting instructions from the LEM/DSO and ISO/TSO, settlements issues, double payments, etc.
IV. THE BTM DER OPTIMIZATION PLATFORM & THE VPP CREATION FOR MARKET PARTICIPATION
In this Section we present a cloud-based DER optimization platform for aggregating, monitoring, controlling and settling IoT smart devices and other DER assets Behind-The-Meter (BTM). The objective is to create Virtual Power Plants (VPPs) and offer their aggregated capacity in wholesale and retail energy markets. Our proposed technology transforms buildings in Grid Interactive Efficient Buildings (GEBs) and offers their aggregated capacity as a VPP to various energy, capacity and ancillary services markets to solve grid problems. It is based on appliance-level service priority tiers and stochastic distributed computing. It coordinates control devices present
in GEBs and provides fast, accurate, and robust demand shaping for groupings of thousands to tens of thousands of devices. Our implemented appliance-level service priority approach meets three major requirements:
- End customers are not directly involved in wholesale or retail auction markets.
- Availability of end-user, controlled demand flexibility in terms of tiers of service provided by individual agreements and the devices they control.
- The coordination problem of determining which devices should consume power at what times is solved through distributed aggregation and virtualized network control.
The proposed DER optimization technology avoids exposing customers to dynamic prices and allows non-coercive participation plans to be formulated intuitively in terms of graceful degradation of the services provided by individual DER assets at the edge of the grid. The customer marks devices using “colors” that correspond to control plans that provide different levels of reliability of services provided by the device. For example, “green” devices can be shut off at any time, “yellow” only at peak power, “red” in emergencies, and “black” can never be shut off. These plans are then executed by the cloud load controller that communicates with an ISO/TSO, LEM/DSO or a regulated distribution company. The implemented load control algorithm then manages instantaneous demand to meet an externally provided demand resource performance target using a feedback loop. The BTM IoT devices switch between states according to the modified Markov model shown in Figure 3 between Enabled (E) and Disabled (D) states probabilistically and from Refractory (R) to Flexible (F) states by a randomized time-out.
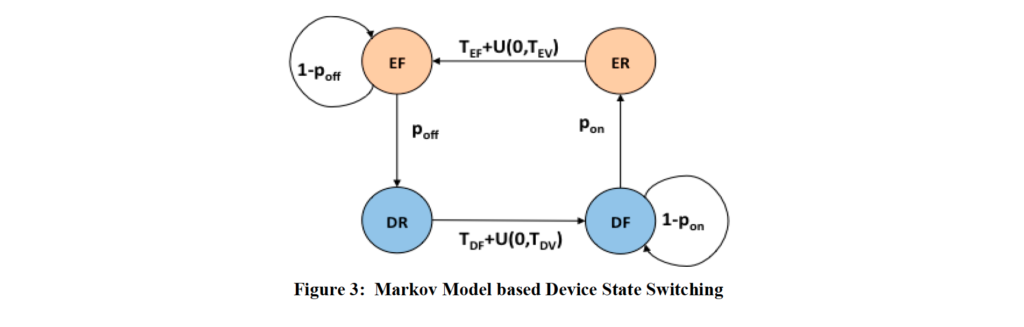
In order to prevent damage to devices and/or customer annoyance, devices must wait through a Refractory period after switching between Disabled and Enabled before they return to being Flexible and can switch again. These combinations give four device states (e.g., Enabled and Flexible (EF)), through which each device moves according to a modified Markov model as shown in Figure 3 randomly from EF to DR and DF to ER (becoming disabled with probability poff and enabled with probability pon) and by randomized timeout from ER to EF and DR to DF (a fixed length of TEF or TDF plus a uniform random addition of up to TEV or TDV). In a system of devices, managed by the cloud-based DER optimization software, at any given time each device (or device behavior mode) is either enabled, meaning that it can draw (or produce) power freely, or disabled, meaning that is has been shut off or placed in a lower power mode.
The state s(t, a) of a device a at time t sums the power demands of the device(s) under its control, and these values are aggregated using a distributed algorithm (e.g., a spanning tree) and fed to a state estimator to get an overall estimate of the true state s(t) of total demand in each state for each color. This estimate is then broadcast to all clients (e.g., by gossip-like diffusion), along with the demand shaping goal g(t) for the next total Enabled demand over all color. Finally, the controller at each client a sets its control state c(t,a), defined as the set of transition probabilities pon,i,a and poff,i,a for each color i. Loads then evolve according to this control state, subject to exogenous disturbances. Devices move from EF to DR with probability poff per round and from DF to ER with probability pon. Devices move from ER to EF by a randomized timeout of TEF + U (0, TEV) seconds, where U (0, TEV) is a uniform random draw from the interval [0, TEV]; the transition from DR to DF is the same, substituting TDF and TDV.
The system comprises a set of n electrical devices, each of which apportions its demand between k priority tiers, where lower numbered tiers are intended to be shut off first and where each tier has its own time constants.
The state s(t, a) of each device a at time t is the magnitude of power demand in each state for each priority tier (e.g.,|EF1, a| is the number of watts of enabled and flexible demand in tier 1 at device a). These values are summed over all reporting devices (some implementations may use sampling for efficiency reasons) using a distributed algorithm and fed to a state estimator to get an overall estimate sˆ(t) of the true state s(t) of total demand in each state for each tier (e.g., |EˆF 1| is the estimated total enabled and flexible demand in tier 1). This estimate is then broadcast to all devices, along with the demand goal g(t) for the next total reduction in enabled demand over all tiers. The controller at each device a uses local information on state, color, and device type to set its control vector c(t, a), defined as the set of transition probabilities pon,i,a and poff,i,a for each tier i. Finally, demands move through their states according to these transition probabilities, subject to exogenous disturbances such as changes in demand due to customer override, changing environmental conditions, imprecision in measurement, etc.
The control algorithm determines the control vector c(t, a) by a stochastic controller formulated to satisfy four constraints, a) goal tracking, b) tier priority, c) fairness and d) device cycling. Since the controller acts indirectly, by manipulating the pon and poff transition probabilities of devices, there are only limited resources for meeting these constraints. At any given time, only the demand in the Flexible states EF and DF is capable of switching between Enabled and Disabled, while Refractory demand must wait for its timer to complete. The controller treats the available aggregated demand flexibility as a “budget” for allocating flexibility to each constraint until either all constraints are satisfied, or all flexibility is spent or reserved for possible future needs. When it is not possible to satisfy all four constraints simultaneously, the controller prioritizes the constraints in order of their importance. First comes goal tracking – the actual shaping of demand to meet power schedules. Second is the soft color priority, which ensures that the contractual obligations with the customer are met. Cycling is last, because it is defined only over long periods of time and thus is the least time critical to satisfy. Each device aggregation independently computes a probability distribution over possible actions and adjusts the state of the devices it controls accordingly, resulting in an aggregate system behavior expected to produce near-optimal system behavior. With each iteration of aggregation, broadcast, and control, the overall system moves toward the target demand, allowing the system as a whole to rapidly achieve any given target demand and closely track target ramp times.
The cloud-based optimization architecture we have implemented at ZOME to build the BTM DER optimization platform is based on a Software as Services (SaaS) framework, called ZED (for ZOME Energy Desk), as shown in Figure 4. It is a M.A.C.H based ecosystem which deploys Microservices, API for all functionalities, is Cloud native, and Headless Frontend agnostic to Backend cloud technologies. It is agnostic to gateway device hardware and provides a Gateway Digital Twin and Digital Lake capability. Further it can scale without setting up additional hardware and infrastructure, resulting in more flexible and scalable operations with dramatically reduced deployment times. The cloud services in the ZED SaaS run asynchronously from each other in every part of the platform including cloud, gateway and webapp. The ZED SaaS platform provides multi-client support for internal (ZOME Gateway) and external (ISO/TSO, LEM/DSO, FDEMS etc.) stakeholders. All the communication between micro-services and 3rd part service follows zero trust and end-to-end security principles. Each stakeholder (i.e., internal or external) of ZED SaaS accesses any resources via revocable access tokens to keep the service access elasticity.
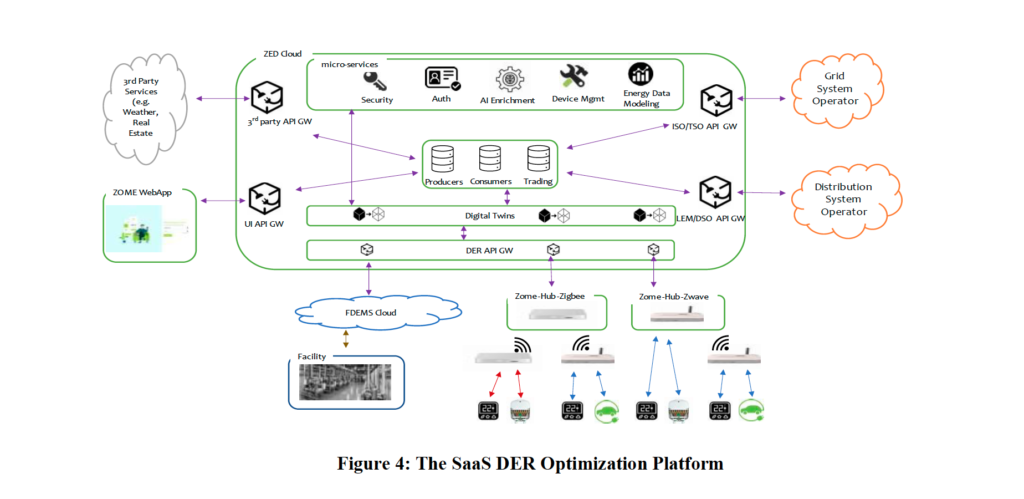
The micro-services keep track of the device state, modify its state as needed, coordinate device communications, including making sure device state is current, ensuring that device is available, logging commands to device and updating the device state to the rest of the system. They also collect available data related to energy usage and data from external services like weather forecasting services and real estate data that could impact energy usage in buildings. Finally, they determine the device state change in control events. Once a control event is created, the microservices examine relevant devices, identify the ones that qualify for state change and determine the new state for chosen devices. Device states can change on a minute-by-minute basis, giving the cloud optimizer the ability to modify device state for curtailment activations and market participation.
The proposed DER optimization platform is ideal for aggregating DERs to unlock their value and bring them into energy markets at scale as VPPs which can emulate dispatchable conventional generators as shown in Figure 5. DER aggregations have system value if they increase the social welfare of the power system and provide value by harnessing economies of scale and managing risks and uncertainties for participating heterogenous DERs with different operating characteristics and control capabilities.
The aggregation of many small controllable load segments of different profile of delivery risks (i.e., different “colors” that correspond to control plans) controlled by the dispatch algorithm in the cloud, (the ZOMECLOUD), mitigates the uncertainty in the energy yield resulting in a VPP with supply reliability characteristics similar to those of conventional power plants. The dispatch frequency of each device is based on subscription contracts with customers which specify the priority of curtailment (delivery risk) vs. compensation. Based on the composition of the contract portfolio and the yield statistics of the VPP load segments, the DER Aggregator constructs a supply function which can be bid into the wholesale and retail energy markets. The proposed DER optimization structure is ideal in allowing DERs individually or in an aggregate to enter into TE transactions, as we’ll elaborate in the next Section.
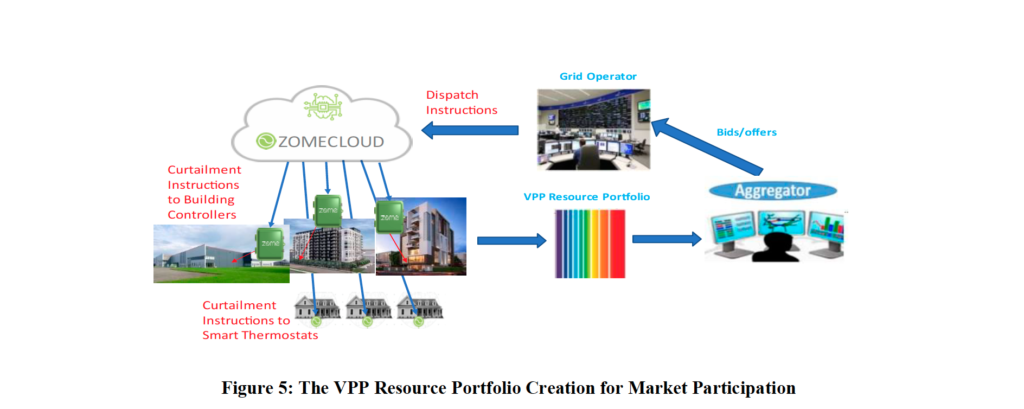
V. THE EMERGENCE OF THE TRANSACTIVE ENERGY MARKET ARCHITECTURE
As we discussed earlier the massive penetration of DERs and the IoT devices in the grid, physically located everywhere, in all shapes and sizes, both in front and behind the meter are fundamentally changing the utility-centric business model. One of the main consequences of this transformation is the emergence of the hierarchical energy market architecture presented above to allow DERs to maximize their value to the grid. Within this evolving market structure, the TE market framework has emerged at the centre of the future grid’s state. TE is a system which consists of coordinated energy participants that use advances in technology, sensing, communications and data analytics to communicate and exchange energy based on value and certain grid reliability constraints. TE uses market–based economic transactions and control functionality for energy trading and sharing among prosumers within an electric power system. In the TE market, energy is becoming a commodity for customers which can be traded among them either on a real time basis or on a predefined schedule. TE systems are expected to revolutionize the relationships between customers, energy companies and the grid. Wholesale energy markets have been operating based on TE principles for more than 20 years; they balance in real-time supply and demand and solve reliability and security problems using price signals produced by the clearing of the spot markets.
However, for the first time now we can build on the emerging multi-tier market structure and apply TE principles to utility distribution systems and business models, and to utility-customer relations – all enabled by the growth of DERs and the IoT revolution. TE transactions include participation in LEM/DSO and ISO/TSO markets along with P2P transactions in low voltage network, energy exchanges between LEMs and other external systems, etc. The LEM-to-LEM exchanges can isolate local systems from the central grid, fostering system reliability for the electrification of everything by decentralizing power generation and reducing pressure on the grid during emergency conditions. Prices mechanisms for non-DSO/ISO transactions could range from a double-sided market in which each participant pays a unique price based on their preferences and local supply and demand conditions (analogous to the stock market) to a proxy price produced by the closest organized market.
The scaling of the TE market will require regulatory changes to a) encourage more investments in distribution and grid connections, b) develop new rate structures and give utilities incentives to adopt more resilient an innovative technologies and b) encourage prosumers diversify backup sources, as well as enable them to sell excel power back to the grid. The investments in the grid cannot be underestimated. For example, in the P2P TE model, each house, apartment, public building, storage facility or RES will have a direct connection between each other. Each individual line should be provisioned with a smart meter to allow for accurate auctioning and tracing each transaction of energy. The TE ecosystem will scale by allowing LEMs to exchange and transact energy between and among each other. Figure 6 illustrates a 5-layer architecture of the TE market. The Regulation Layer deals with market regulations, laws, standards and policies for information security and data protection. The DER Device Layer includes the IoT devices, the DER assets and their platform for the TE transactions. The Communication Layer is deployed by the DERs in the second Layer to communicate with the downstream Layers with specific requirements on reliability, security, latency and bandwidth. The market Layer deals with market applications, market clearing, market pricing, databases, data analytics, etc. and communicates with the Distributed Layer. The Distributed Layer includes public main nets and private permissioned sidechains to record the TE transactions and LEM pricing and ensure energy and economic validation as we explain below.
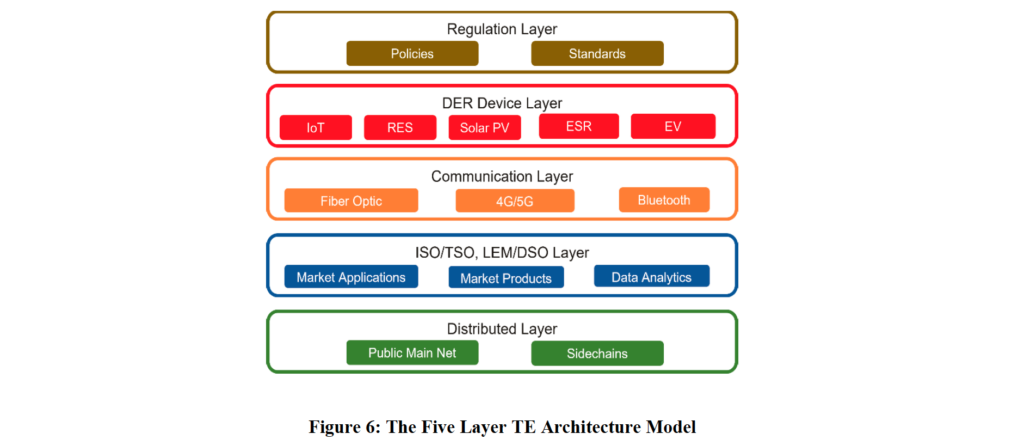
The TE market model we have designed, and we are in the process of implementing, runs on blockchain capable of scaling to any level within a grid. It consists of multiple permissioned TE sidechains, as part of the global Ethereum Public Main network that encrypt and record transactions for energy in LEMs/DSOs and ISOs/TSOs, and P2P transactions, through a secure international ERC-20 Token and settlement standard. The TE sidechains can be deployed in worldwide regional markets and can scale modularly as more participants and authorities are added to the platform. Each TE sidechain can be transacted in a LEM where participants are located. By controlling a digital wallet, they can participate in energy loyalty and reward token program incentives. Collectively these regional sidechains are linked to a universal TE Public Main Net, which creates a scalable and truly global TE Network as illustrated in Figure 7. Authorized and authenticated TE participants connect to the TE sidechains to transact via smart energy and capacity contracts that immutably document transaction processes. The TE sidechain platform design allows for multiple types of smart contracts to be created in an ongoing basis as more services are added to the TE platform. These smart contracts run on blockchain validation nodes, called “TE Validators,” that establish the consensus mechanism as the core principle of our TE blockchain platform.
The TE sidechains interconnect to third party trusted oracles (e.g., Meter Operators, utility meter data repositories) and other data service layers (e.g., AI/machine learning data forecast and analysis) to create an energy service layer that collects data, including “big data,” from DSOs/ISOs and/or energy service providers (e.g., DER Aggregators). The interface is accomplished with APIs that are part of the TE platform. The immutable distributed energy data is stored in the Distribution Energy Data Store (DEDS) database and the cryptographic data hashes are appended to each sidechain to store cryptographic data from energy transactions. The DEDS resides in any TE node and is part of the distributed file-sharing network to guarantee data integrity, privacy, and security. The API interfaces with the TE network using cryptography security protocols to authenticate the meter readers as a trusted data source to be error-checked and logged into the system.
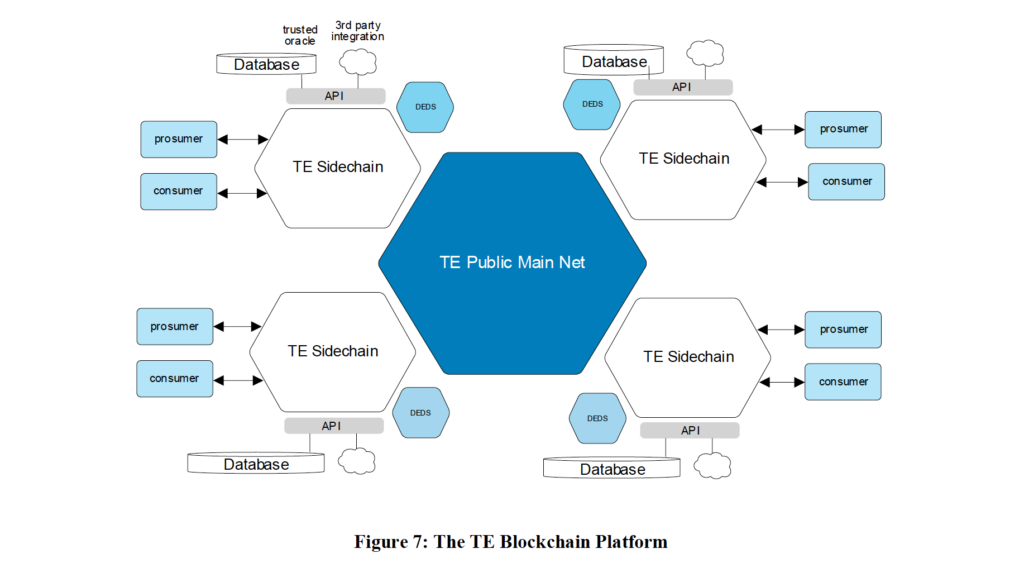
The TE platform also interfaces with fiat and other cryptocurrencies via cryptocurrency exchange gateways and provides loyalty rewards to all local permissioned blockchain sidechain TE LEM platforms via Ethereum wallets. In the permissioned TE sidechain, TE participants can transact (buy and sell) energy in P2P transactions or DSO/ISO energy markets and earn Tokens using their digital wallets. These Tokens are issued by the Ethereum TE Public Main Net and used by the sidechain energy participants as a mechanism to earn reward/loyalty points that later can be exchanged by any fiat, cryptocurrency, or be held as a storage investment financial instrument. The Token is designed with mechanisms in place to support its value through the process of periodic “token burning” by permanently removing a portion of tokens from the total circulation supply to counterbalance inflation depending on the growth rate of the WEC network. By automatically adjusting in both directions the total number of Tokens in circulation, the TE reward proprietary algorithm maintains a relatively stable value for Tokens at their time of issuance relative to the state of the TE network.
In closing it is important to note that a critical factor for a wider adoption of the TE market is the development of standards in several areas including, consensus algorithms, governance, interoperability, smart contracts, etc.
VI. SUMMARY AND CONCLUSIONS
The massive penetration of DERs and the IoT devices in the grid are fundamentally changing the utility-centric business model. One of the main consequences of this transformation is the emergence of the hierarchical energy market architecture under which DERs can voluntarily participate simultaneously in multiple-tier hierarchical markets, namely ISOs/TSOs, DSOs/LEMs, BTM and TE markets. Specifically, the TE approach by transforming the grid to a decentralized, digitized, democratized and resilient network, it can offer many potential benefits, both to individuals and to society. However, the developed TE technologies must ensure transparency, choice, and ease of use to prosumers. At the same time, the operators who control and manage the electrical grid must be able to use these technologies while continuing to provide electricity safely, reliably, and efficiently.
For Further Reading
[1] Alex Papalexopoulos, Jake Beal and Steven Florek, “Precise Mass Market Energy Demand Management Through Stochastic Distributed Computing,” IEEE Transactions on Smart Grid, IEEE Power & Energy Society, Vol. 4, No. 4, Dec. 2013.
[2] Alex Papalexopoulos, Rod Frowd and Alexios Birbas, “On the development of Organized Nodal Local Energy Markets and a Framework for the TSO-DSO Coordination to be presented at the 20th Power Systems Computation Conference (PSCC), Porto, Portugal, June 29-July 3, 2020.
[3] U. Cali, C. Lima, X. Li and Y. Ogushi, “DLT / Blockchain in Transactive Energy Use Cases Segmentation and Standardization Framework,” 2019 IEEE PES Transactive Energy Systems Conference (TESC), Minneapolis, MN, USA, 2019, pp. 1-5.
BIOGRAPHY
Alex D. Papalexopoulos is CEO of ECCO International and CEO & Chairman of the Board of ZOME Energy Networks.